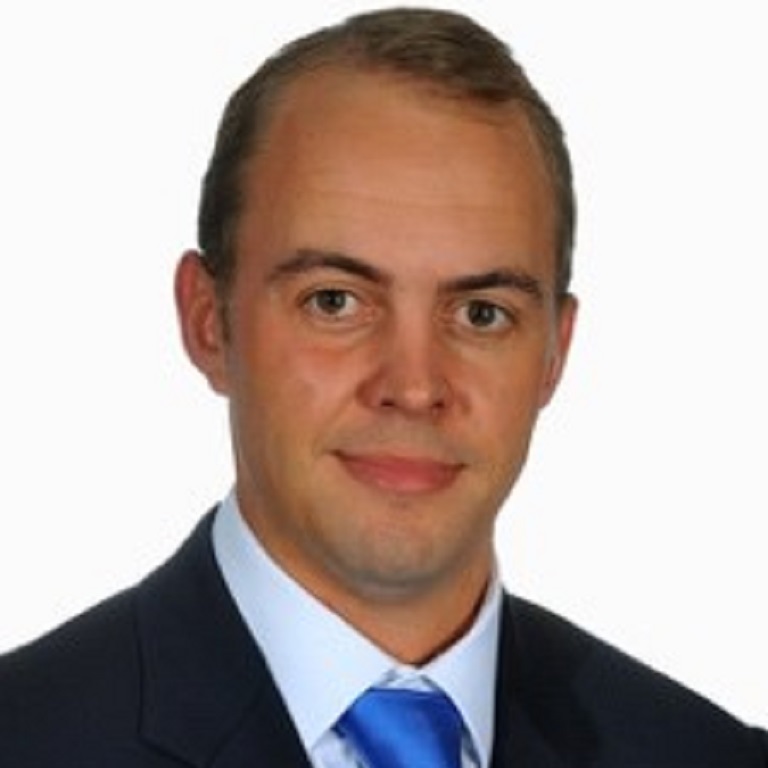
George Baldwin
Climate Resilience and Strategy Advisory Leader, UK, and Climate Centre of Excellence Co-Leader
-
United Kingdom
In the last few years, the collective realisation that climate change is not a possibility, but an emerging operating reality, has posed the business and financial community with a new question: How do you invest confidently in a world where, within the investment horizon, a changing climate can impact the viability of an asset or an entire business?
The climate agenda has found its way to the boardroom of many organisations, for both prudent risk management and increased regulatory pressures. Whether it’s mandated stress tests for banks or public reporting — such as via the Task Force on Climate-related Financial Disclosures (TCFD) framework — for others, it boils down to the need to ingest new and complex climate data, connect that data to existing functions and models, and to do so at pace.
For many, it represents a greater reliance on third-party data and models than is natural or comfortable based on historic risk management approaches. This does not mean climate models themselves should be taken on faith, but rather that understanding them and what good looks like is becoming a new fundamental and imperative for leadership.
While climate modelling in this context is relatively new, its precursor, natural catastrophe modelling, is a well-established industry. Primarily focused on serving the insurance world for present-day pricing and capital modelling, the distillation of physical risk into an expected view of loss ”risk today”’ means a lot of the component parts of “risk tomorrow” are well tested and well audited. (It also has the helpful bonus that if an insurer is likely to use the same model, it should come to similar conclusions on a property’s risk profile, for example.) And, while a 30- to 100-year climate model cannot be audited the same way against present-day outcomes, one should expect common underpinnings of the same quality, for example:
Bolting on the climate-adjusted aspect of risk is far from trivial business, but equally, no trend analysis is meaningful without a clear and reasonable view of risk today as a starting point.
All the above focuses on how to assess an individual property, but for this kind of modelling it’s very difficult to produce a meaningful portfolio-level answer any other way. So whether modelling a mortgage book, a real estate investment trust, or the operating assets of a company, the specific locational context of each asset needs careful consideration.
To bring this to life, we can compare this bottom-up approach to the aspiration of many organisations looking to create postal code-level databases of climate risk. The smart way to do this is to aggregate climate data on the basis of the individual property risk profiles in the postal code; the alternative is to create an average of some kind over the land mass, regardless of where properties are located, which will likely skew the view of risk (for example, no one would build a house in the river that flows through the middle of the postal code).
Similarly, properties built in an area which is known to be riskier today should be built to a higher standard of resilience to that peril. This can be as simple as using more nails on roof tiles in windy areas, or as dramatic as building houses on stilts in flood-prone areas. One can therefore readily see the dangers of under- or over-reporting risk that arises without understanding how risk and property design interact.
It’s very important for an organisation to arrive at its own view of what climate risks need to be modelled, rather than to accept a data vendor’s view, which will likely align to its own product.
Several inputs can be useful here:
Notably, for property portfolios, it’s common for flood-related risks, wind/hurricane, and wildfire to drive the majority of direct impacts, while extreme heat, water stress, and drought cause longer-term indirect impacts, such as business disruption (down days or adjusted work hours).
There will likely be overlap between an organisation’s priority list and what is available from the climate model community, but not a perfect fit. Presently, there are few climate-adjusted landslip or tornado models available, for example. That said, virtually all climate model vendors have ambitious roadmaps for augmenting capability: given a typical vendor will work with a client for five-plus years (as the integration into processes can run very deep), it’s as important to understand (and potentially influence) these roadmaps so as to understand their current capabilities. We have included in the box out some potential questions to ask regarding commonly sought peril models.
No two vendors produce the same suite of outputs. Some focus more on a score system, which promises simplicity, while others offer an array of data points seeking to describe the climate-risk distribution. For investment modelling, we tend to recommend working with the latter. Given the non-linear relationship between financial stress and credit-worthiness, knowing whether an organisation is exposed to frequent, nuisance-level risk, or highly unlikely catastrophic risk (the latter typically being more insurable than the former) is important. For this reason it’s important to focus on data structured in return periods or similar and avoid score-based averages, which invariably reduce granularity.
We have typically found that organisations create their own score-based system from this data in order to aid decisioning. The difference here is that the simplification is owned and understood in-house rather than being at the behest of the vendor. This also has the advantage of the organisation being less tied to the data architecture of a particular vendor — return-period-structured data is fairly common, whereas scores are always bespoke.
It’s worth noting that virtually all vendors focus on property damage rather than business interruption risk as the latter requires much more information about the nature of the enterprise, the criticality of various sites to their ability to generate revenue, and so forth. It’s perfectly possible for an investment house to build this up using a vendor’s damage data as an input, but few vendors can meaningfully provide this answer outside in. The same is true for value chain analysis: if this is not first mapped by the investment house, it’s unlikely that the data vendor would be able to model it meaningfully.
We have also found that how vendors choose to characterise their product varies wildly:
Again, an organisation’s use cases will dictate how important each of these dimensions are. Ease of execution might be appropriate for experimental use or very high level reporting, while the quality and granularity of the model will likely matter more when making active investment decisions.
No physical risk vendor will claim to have perfect foresight, and the data can be inherently noisy. Auditing a vendor’s approach is the best source of comfort, and is best achieved through the following:
Where practical and helpful, you should back-test present-day risk for certain perils and geographies against real-world outcomes. You should expect a correlation between major recent events and locations, which would should show as a higher risk in the model. This is best undertaken by an independent party, though the vendor should have an internal process equivalent to this.
The outputs of these models are useful across scenario analysis and reporting (such as the stress tests and TCFD reporting mentioned above), internal testing of existing risk positions, and front-book decision making. Ideally, an organisation will use the same model across all these exercises (and across business units, such as debt and equity investments) in order to ensure consistency of appetite.
The final step to consider is how to increase the resilience of the organisation’s assets. This can mean many interventions with the occupier, such as:
The above suggestions represent better overall economic outcomes than rendering assets and businesses stranded, but may require a step-change in engagement from certain investors.
Depending on both the timeframe of the physical risk profile evolution and the length of investment, many changes can be encompassed into existing operational risk management processes with limited disruption. The key is to bring forward the analysis and communication of risk to maximise the window for resilience building and adaptation.
Physical risk data modelling is a rapidly evolving technical area. It’s small wonder that large investment organisations have on-boarded staff fluent in the design and use of these models to ensure full value is extracted from them.
At the same time, they will only ever tell a subset of the physical risks a location or entity might face. They are not designed, for example, to conduct what-if analyses of how a commercial location might be affected by nearby residential abandonment arising from physical risk, and none will tell you directly how to keep a property insurable in adverse circumstances (thus preventing technical default by breach of covenants).
Still, their shortcomings are not a reason to delay their integration into your organisation to safeguard against adverse selection, better understand long-term risk, and begin to build capability for the realities of climate change.
Perils (subset only shown)
Features
Climate Resilience and Strategy Advisory Leader, UK, and Climate Centre of Excellence Co-Leader
United Kingdom
Physical Risk Modelling Lead, Climate Resilience & Strategy, Marsh Advisory UK
United Kingdom